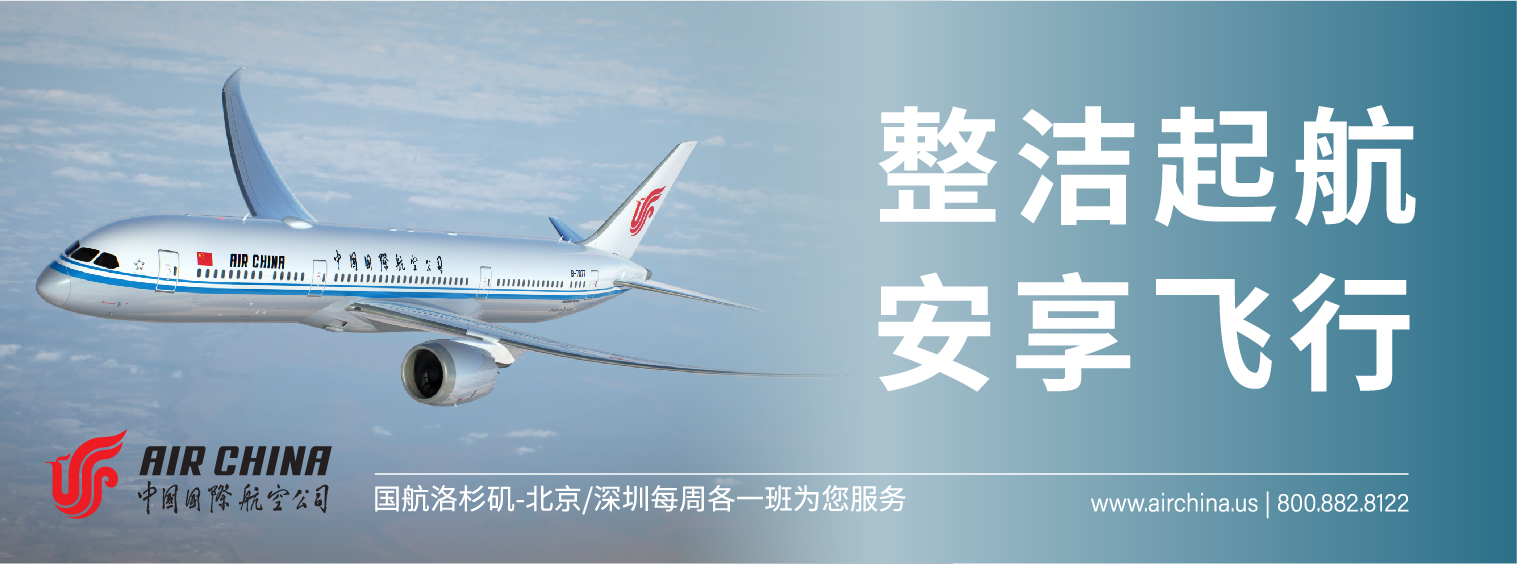
“味在西关”小林美食漫画展在广州开展
4月26日,“味在西关”小林美食漫画展在广州市荔湾区开展,吸引参观者观展拍照。“小林漫画”主创林帝浣...
2024-04-27阅读(9974)赞 (21)
4月26日,“味在西关”小林美食漫画展在广州市荔湾区开展,吸引参观者观展拍照。“小林漫画”主创林帝浣...
2024-04-27阅读(9974)赞 (21)
当地时间4月25日,抗议者在美国加州斯坦福大学参加示威活动。连日来,数所知名美国高等学府爆发示威,要...
2024-04-27阅读(11698)赞 (10)
4月26日,工作人员打跳推介昆明。当日,“花‘漾’昆明”2024昆明文旅赏春宴暨五一旅游推介会在云南...
2024-04-27阅读(9480)赞 (29)
4月26日下午,中国国家主席习近平在北京人民大会堂会见美国国务卿布林肯。 未经允许不得转载:城市新闻...
2024-04-27阅读(8238)赞 (25)
4月26日,2024年成都世界园艺博览会在四川成都开幕。本届博览会以“公园城市 美好人居”为主题,主...
2024-04-27阅读(9599)赞 (42)